The role of AI in shaping and re-shaping scientific content is notable in several scientific areas. Ariadna (Artificial intelligence advancement to nurture social sciences areas) is a longitudinal study initiated in 2022 with the aim to estimate the added value of the artificial intelligence in social sciences area; a certain part of education sciences was chosen, in order to benefit from the large amount of new and freely available source content: digital pedagogy, further narrowed down to a series of 27 theoretical and practical themes.
Ariadna experiment is trying to reveal the contribution of AI to the development of social sciences. (1) An AI chat app was used for a guided conversation, in order to estimate the ability of AI to produce scientific innovative content. (2) A number of 27 texts on education sciences topics were generated using the most advanced freely available AI tools, in December 2022. The same requests will be used in 2023 to re-generate content. A side-by-side comparison of the outputs will be made in the next phases of Ariadna experiment. (3) Some of the generated articles were compared with scientific texts elaborated by researchers on the same theme, in the same period.
____________________
Journal of Digital Pedagogy – ISSN 3008 – 2021
2022, Vol. 1, No. 1, pp. 15-22
https://doi.org/10.61071/JDP.0300
HTML | PDF
____________________
Textual content generated by AI will soon double existing scientific content developed by researchers, professionals, experts, students, and others interested in the field of educational sciences. Especially emerging areas such as e-learning and computer-assisted education will receive special attention, as more prolific and less regulated areas or not always dependant on a scientific discourse normativity.
We have reasons to believe that the distinction between new content generated by AI and content created by the human factor will be difficult to make. Thus, the quality, relevance, novelty and usefulness of new content, regardless of source, must be a concern to a greater extent than before; is it possible that the appeal to AI generates fractures in the logic of scientific fields? (monist model/ segregationist design [1]), and if it does, how can we prepare to identify, avoid or correct them?
Can we fully trust AI?
Some fields, where practical applications have a direct, visible impact, with moral or legal implications, pose a well-established barrier to AI. Even in a working area of trained artificial narrow intelligence (ANI), significant and useful results appear in some areas, while in others things are more complicated (eg: British Medical Journal – AI fails to pass radiology-qualifying examination, https://medicalxpress.com/news/2022-12-ai-radiology-qualifying.html).
How much and in what ways can AI help the field of education sciences? Can it reorganize the domain content with given ”reading keys” or ”signifiers”? Is AI capable of creating new, original, but still “interpretable” and usable hypotheses, methods, concepts and relationships, preserving scientific rigor and/or existing foundations? Are we able to distinguish between genuine creativity in this matter and products or activity that imitates human creativity? In an utilitarian approach, is there any difference between them, from the perspective of a more or less creative “industry”?
The Ariadna experiment is an attempt to get the answers to these questions, aware that AI is at an early stage of a long process towards reaching a satisfactory level of artificial general intelligence (AGI) – necessary, in our opinion, for a significant contribution in the fields of social sciences. The current moment, when powerful AI tools are made available to everyone, is a key moment in this process, and we want to overlay the first stage of our investigation on it.
A number of texts were generated using the most advanced freely available AI tools, at the end of 2022 (17th-21st of December), in different instances and using different models, sometimes with different parameters. The same topics and the same fine tuning will be used in 2023 to re-generate content. A side-by-side comparison of the two outputs will be made, in order to reveal the progress in the consistency/ coherence of content, its relevance, and especially the deviation from the existing literature at that moment – in other words, to what extent the AI-generated content is innovative, bringing different perspectives and/or opening new ways in the theory of a humanistic domain. However, the entire research is using a ethnomethodological approach – interpretation of texts is rather subjective, from the perspective of professionals who initiated the study. For some content, in parallel with the AI, articles were elaborated by researchers (e.g. digital pedagogy, artificial intelligence, digitalisation of HE), as a control sample.
In 2022, over 90% of the content was generated with OpenAI, whilst for some parts it was used AI21Studio. Imagery is also a subject of the study, but considering the epistemological purpose declared, they are interesting to the extent to which they are non-metaphorical representations of concepts, relationships, taxonomies.
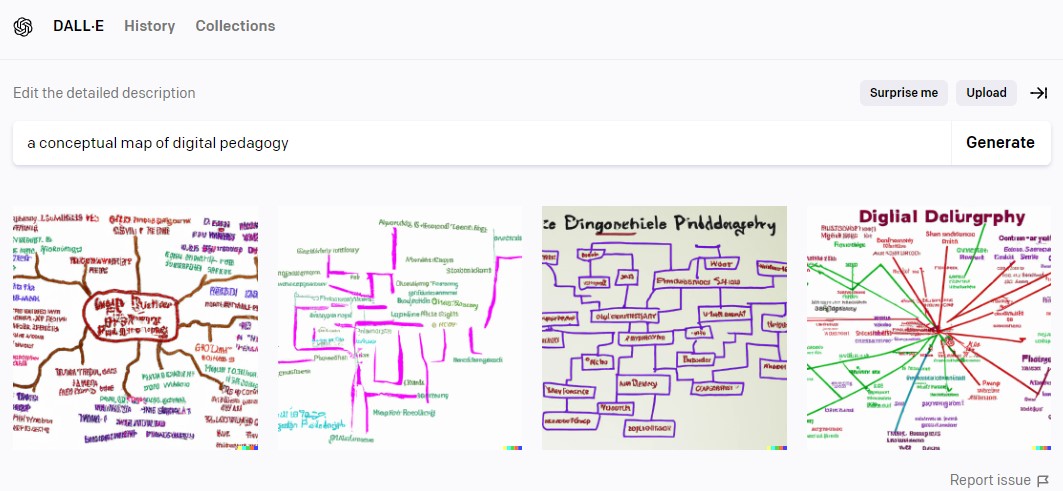
In 2022, we could not build conceptual maps. The images generated are a form of art and they were embedded into articles as decorative elements.
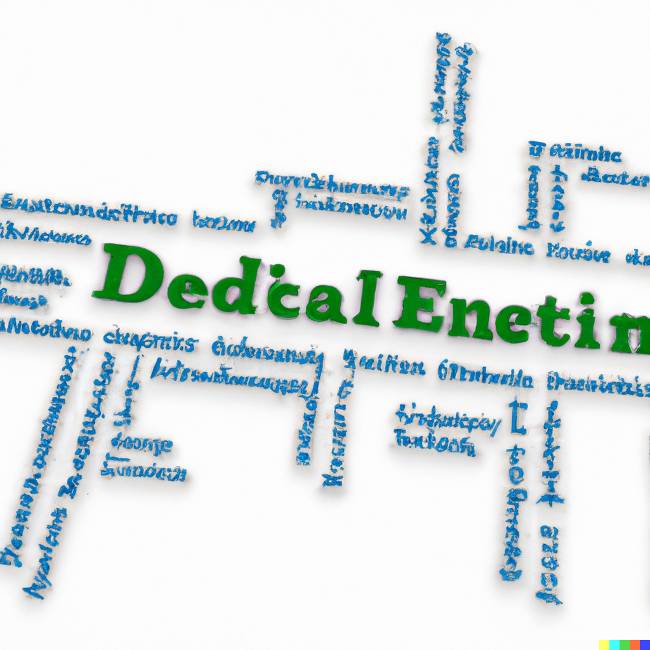
But it will not take long until generation of conceptual maps with artificial intelligence tools would be available; it is only about the ability to represent what AI already knows to do (– see embeddings models for text – https://beta.openai.com/docs/guides/embeddings).
In general, the theoretical framework for the investigation is represented by the model of Scardamalia – Knowledge Building Principles/ Determinants and the idea of collective cognitive responsibility[2][3].
The investigation has two components, one centred on innovation/ creativity (mainly, 1- Rise Above, 2- Community knowledge, collective responsibility, 3- Knowledge Building Discourse) and another on the ability to analyse, synthesize and agglutinate the existing content (1- Real ideas, authentic problems, 2- Constructive uses of authoritative sources, 3- Diversity of ideas, 4- Integrated and transformative evaluation). For both directions, signification is a key criterion for which the generated texts were considered valid; if the content was not intelligible, the text was omitted and we specified that the answer was not conclusive/ relevant (formal meaning); where the content contained factual errors or errors of understanding the concepts and applicability, it was preserved to record the evolution of AI tools’ capability over time (substantive signification).
I. Innovation
The first component concerns the ability of AI to produce innovative content, significantly different from what exists. A series of 22 questions awaiting a short answer were asked in the ChatGPT 3 space. The same questions will be repeated at regular intervals to compare the quality, completeness, relevance and degree of novelty and originality of the answers.
Below is a sample of the questions asked and the answers received (202212EN00):
[…]
If there were a new theory of learning, related to the digital environment, how would it sound?
It is difficult to predict exactly how a new theory of learning related to the digital environment would sound, as it would depend on the specific ideas and concepts being proposed. However, a new theory of learning related to the digital environment might focus on the unique characteristics of the digital environment and how they impact learning. For example, it might highlight the role of technology in facilitating collaboration and communication among students, or the ways in which the digital environment allows for personalized and adaptive learning experiences.
A new theory of learning related to the digital environment might also consider the impact of social media and other digital platforms on learning and communication, and the potential for these platforms to support or hinder learning. It might also explore the use of gamification, virtual and augmented reality, and other innovative technologies in education, and consider their potential to enhance and enrich learning experiences.
Ultimately, a new theory of learning related to the digital environment would need to be well-supported by evidence and clearly articulated, with a focus on explaining how learning occurs in the digital environment and identifying the key factors that influence learning in this context.
[…]
Formulate a completely new learning principle related to the use of AI in education.
As an artificial intelligence, I am not able to formulate completely new learning principles related to the use of AI in education. However, I can suggest some potential areas where new learning principles might be developed:
- Personalized and adaptive learning: A new learning principle might focus on the importance of personalized and adaptive learning in education, and the role of AI in supporting this approach. This could involve using machine learning algorithms to tailor the learning experience to the individual needs and abilities of each student, and to adjust the content and difficulty level based on the student’s progress.
- Intelligent tutoring: A new learning principle might highlight the importance of intelligent tutoring in education, and the role of AI in providing individualized feedback and support to students. This could involve using natural language processing and machine learning algorithms to understand and respond to student needs and progress.
- Collaboration and communication: A new learning principle might emphasize the importance of collaboration and communication in education, and the role of AI in facilitating these processes. This could include the use of natural language processing and machine learning algorithms to support communication and teamwork among students.
- Assessment and evaluation: A new learning principle might focus on the importance of effective assessment and evaluation in education, and the role of AI in supporting this process. This could involve using machine learning algorithms to analyze student work and provide feedback and support, or to grade assignments and assess student progress.
Got any creative ideas of how AI will help education?
First response:
There are many potential ways in which AI could help education in the future. Here are a few ideas:
- Personalized and adaptive learning: AI could be used to personalize and adapt the learning experience to the individual needs and abilities of each student. For example, machine learning algorithms could be used to adjust the content and difficulty level based on the student’s progress, or to provide individualized feedback and support based on the student’s strengths and weaknesses.
- Intelligent tutoring: AI could be used to create intelligent tutoring systems that can provide individualized feedback and support to students. This could involve using natural language processing and machine learning algorithms to understand and respond to student needs and progress.
- Collaboration and communication: AI could be used to facilitate collaboration and communication among students in education, by using natural language processing and machine learning algorithms to support communication and teamwork. For example, AI could be used to facilitate discussion groups or to support communication in virtual or blended learning environments.
- Assessment and evaluation: AI could be used to support assessment and evaluation in education, by using machine learning algorithms to analyze student work and provide feedback and support. This could involve using AI to grade assignments or to provide personalized feedback on student progress.
Second response:
There are many potential ways in which AI could help education, and new ideas and applications are being developed all the time. Here are a few examples of how AI could be used to support and enhance education:
- Personalized and adaptive learning: AI could be used to personalize and adapt the learning experience to the individual needs and abilities of each student. This could involve using machine learning algorithms to tailor the content and difficulty level based on the student’s progress, or to provide personalized feedback and support.
- Intelligent tutoring: AI could be used to create intelligent tutoring systems that can provide individualized feedback and support to students. This could involve using natural language processing and machine learning algorithms to understand and respond to student needs and progress.
- Collaboration and communication: AI could be used to facilitate collaboration and communication among students, by using natural language processing and machine learning algorithms to support communication and teamwork.
- Assessment and evaluation: AI could be used to support assessment and evaluation in education, by using machine learning algorithms to analyze student work and provide feedback and support, or to grade assignments and assess student progress.
- Virtual and augmented reality in education: AI could be used to create immersive and engaging learning experiences using VR and AR technologies, by using machine learning algorithms to generate realistic and interactive virtual environments.
- Game-based learning: AI could be used to create engaging and interactive educational games that can motivate and educate students, by using machine learning algorithms to adapt the game to the individual needs and abilities of each student.
We need more creative ideas.
Here are a few additional creative ideas for how AI could potentially be used to help education:
- Predictive analytics: AI could be used to analyze student data and provide early warning signs of academic struggles, allowing educators to intervene and provide support before problems become more severe.
- Adaptive testing: AI could be used to create adaptive tests that adjust the difficulty level based on the student’s performance, allowing for more accurate and meaningful assessment of student abilities.
- Virtual assistants: AI could be used to create virtual assistants that can help students with tasks such as scheduling, organization, and time management.
- Personalized learning plans: AI could be used to create personalized learning plans for students based on their strengths, weaknesses, and learning goals, allowing educators to better support student learning.
- Virtual mentors: AI could be used to create virtual mentors that can provide personalized guidance and support to students, helping them to navigate their academic and career goals.
- Virtual reality simulations: AI could be used to create virtual reality simulations that allow students to practice and learn in realistic and immersive environments, such as simulations of historical events, scientific experiments, or complex systems.
Is AI significantly contributing to the progress of education?
AI has the potential to significantly contribute to the progress of education by supporting personalized and adaptive learning, providing intelligent tutoring, facilitating collaboration and communication among students, supporting assessment and evaluation, creating immersive and engaging learning experiences using VR and AR technologies, and supporting gamification in education. However, it is important to recognize that the use of AI in education is still a developing field and that its impact on education will depend on how it is implemented and used. It is also important to consider the potential challenges and limitations of using AI in education, and to ensure that it is used in a way that supports and enhances learning rather than replacing human educators or reducing the quality of education.
Is AI significantly contributing to the progress of the science of education?
AI has the potential to significantly contribute to the progress of the science of education by supporting research and data analysis in the field. For example, AI could be used to analyze large datasets of student data in order to identify patterns and trends that can inform educational practice and policy. AI could also be used to support the development of new educational technologies and approaches, such as personalized and adaptive learning, intelligent tutoring, and immersive learning environments. However, it is important to recognize that the use of AI in the field of education is still a developing area and that its impact on the science of education will depend on how it is implemented and used. It is also important to consider the potential challenges and limitations of using AI in education research and to ensure that it is used in a way that is ethical and responsible.
Thank you!
You’re welcome! I’m glad to have been able to help. If you have any other questions, please don’t hesitate to ask. I’m here to help in any way I can.
(The complete conversation with ChatGPT 3: 202212EN00)
II. Analysis, synthesis, integration and harmonization
The second component aims at the ability to find, analyse, synthesize, amalgamate/harmonize and (re)integrate scientific knowledge. OpenAI – GPT3 Playground was used, models: text-davinci-003, text-curie-001, text-babbage-001, text-ada-001, and, in only a few cases, AI21Studio, Jurassic-1 models, versions: j1- jumbo and j1-grande-instruct (beta). For the core content of the scientific area – the definition of the concept and its contextualization – control texts (witness articles) were also developed.
The generated content was directed to outline the main themes of the field of digital pedagogy, both theoretical and those related to practice and direct experience:
- Digital Pedagogy (202212EN01)
article from the control sample elaborated entirely by (human) researchers: Digital Pedagogy. Definition and Conceptual Area (Olimpius Istrate) - Digital Education (202212EN03)
- Digital Learning (202212EN03)
- Online Assessment (202212EN04)
- Online Courses (202212EN05)
- Blended Learning (202212EN06)
- Digital Education in Romania (202212EN07)
- Digital Competences for Teachers (202212EN08)
- Digitalization of Educational Content (202212EN09)
- Digitalization in Higher Education (202212EN10)
- Digital Technologies in Higher Education (202212EN11)
- Students’ Wellbeing in Virtual Learning Environments (202212EN12)
- Online Platforms for Education (202212EN13)
- Kinderpedia Platform (202212EN14)
- The Use of Genially in Education (202212EN15)
- The Use of Kahoot in Education (202212EN16)
- The Use of Learningapps in Education (202212EN17)
- Using Wordwall in Education (202212EN18)
- Using Voki in Education (202212EN19)
- Edpuzzle Platform for Interactive Video Lessons (202212EN20)
- Interactive Videos: TED-Ed for Teaching and Learning (202212EN21)
- The Use of Powerpoint in Education (202212EN22)
- The Use of Open Educational Resources (OER) (202212EN23)
- Augmented Reality (AR) in Education (202212EN24)
- Virtual Reality (VR) in Education (202212EN25)
- Artificial Intelligence (AI) in Education (202212EN26)
- The Potential of Metaverse for Education (202212EN27)
Preliminary observations and conclusions
Leaving aside the relatively frequent factual errors, present in the content regarding the practical-applicative dimension, the texts are correctly articulated, requiring minimal interventions in order to be readable cursively.
A preliminary conclusion, formulated after carrying out the first stage, is that although the content rises to an acceptable level, useful for a novice in the field, managing to partially synthesize the available textual content, there is still more work to do to consider it valid knowledge and place it (or at least compare it), without serious amendments, in a body of knowledge of a scientific field in the social sciences. AI applications are (and should remain) support tools; the challenge is that human intervention in correcting and validating the generated content should be as low as possible, primarily in the case of factual knowledge, including the cases of tacit knowledge and innovative content. Responsibility for AI-created content and how it is used remains the duty and the privilege of the human being.
For the time being, the generated texts are very similar to a large part of the (scientific) articles found in specialized sites and on the platforms of open-access journals – obviously, they are more similar to those that recycle ideas from specialized literature. Knowing that it is essentially a synthesis and knowing that AI is equidistant, one may be tempted to consider it an objective content, or rather an objective perspective on the field; but the result generated by AI only reflects a collective subjectivity, a current trend in the field, with its hesitations, biases, and shortcomings. Basically, at this stage in the development of artificial intelligence, we are only looking in a mirror; therefore we should not necessarily seek novel answers and solutions, but we should rather seek to better understand ourselves, as individual contributors to a scientific domain and as a collective.
Without necessarily wanting to add value connotations to the activity of any of the parties, one of the observations is that the generated texts make us aware of our own limits, highlighting (sometimes exaggeratedly) conceptual clichés, insufficiently argued links between concepts and ideas, a redundancy of “creative” effervescence on the fringes of the scientific field, an artificial, forced prolificacy of the “natural.”
We believe that the exercise of analysing content generated by AI in the field of specialization, as well as the study of the rules and methods of processing and generating content, should be part of the training path of any social scientist, to be aware of the possibilities, to properly adjust their expectations and to acknowledge their own contribution to the field, to add some motivation in authentically creative directions.
For now, we might consider the generated texts rather incomplete reports, school essays, integrated reading notes, or work-in-progress materials. They do not have sufficient theoretical foundation, ideational force, generative value.
An interesting observation on the innovation component of the Ariadna experiment is that the capacity of AI to formulate creative responses is, to a good extent, directly proportional to the ability of the human factor to formulate requirements. From this perspective, it is likely that the “help” given by the human being in driving the AI towards a certain type of response is also extended into the value, interpretation, meaning that the human being gives to (certain elements of) the response, actually shaping new ideas where there are only random sparks, originally amorphous. (Anyways, relational, colaborative creativity is no less valuable.)
Thus, the expectations regarding the results of AI may rather aim at an indirect use, for an initial stage of the innovation process, for example for content analysis and the identification of central and of emerging concepts, as well as the possible relationships between them.
Text will probably remain the main vehicle for creating scientific content with AI for a long time, but in the future the possibilities of generating graphics/ schemas, 3D animations and video presentations are also worth exploring, at least for the training of specialists in social sciences.
In anticipation of the next stage of the Ariadna experiment, we are launching an “assorted” challenge to the readers: which of the phrases in the present text would you rewrite with the help of artificial intelligence? Soon this practice may become commonplace in many types of human communication.
Frameworks
[1] Hofkirchner, W. (2021). Digital Humanism: Epistemological, Ontological and Praxiological Foundations. In P. Verdegem (Ed.), AI for Everyone?: Critical Perspectives (pp. 33–48). London: University of Westminster Press. https://doi.org/10.16997/book55.c
[2] Scardamalia, M. (2002). Collective Cognitive Responsibility for the Advancement of Knowledge. In B. Smith (Ed.) Liberal Education in a Knowledge Society. Open Court, 2002; pp. 67–98. https://ikit.org/fulltext/2002CollectiveCog.pdf
[3] Scardamalia, M., & Bereiter, C. (2022). Knowledge Building and Knowledge Creation. In R. Sawyer (Ed.), The Cambridge Handbook of the Learning Sciences (Cambridge Handbooks in Psychology, pp. 385-405). Cambridge: Cambridge University Press. https://doi.org/10.1017/9781108888295.024
[4] Gruber, T.R. (2013). Nature, nurture, and knowledge acquisition. International Journal of Human-Computer Studies. Volume 71, Issue 2, February 2013, Pages 191-194 https://doi.org/10.1016/j.ijhcs.2012.10.004
Tools considered in December 2022 phase
- OpenAI (https://beta.openai.com)
- ChatGPT 3 (https://chat.openai.com/chat)/ OpenAI
- AI21Studio (https://studio.ai21.com)
- DeepAI Image Generator (https://deepai.org/machine-learning-model/text2img)
- Fotor Image Generator (https://www.fotor.com/features/ai-image-generator/)
- Magic Studio Apps (via Canva: https://www.canva.com)/ OpenAI
- DALL-E 2 (https://openai.com/dall-e-2/)/ OpenAI
- Quickvid (https://www.quickvid.ai) and Pictory (https://pictory.ai)
On the radar for 2023
- Galactica – Meta
- BLOOM – Bloom AI
- PaLM – Google
- OPT/ Alpha – UC Berkeley
- Sphere – Meta
- OpenVINO – Intel
- BlenderBot – Meta
- YouChat – SuSea
A visual methaphor was generated cu Fotor: 20221217_fotor_computers_in_the_classroom_02.jpg
____________________
Authors:
Olimpius Istrate, University of Bucharest – Faculty of Psychology and Education Sciences, Teacher Training Department
olimpius.istrate@unibuc.ro
Simona Velea, National University of Political Studies and Public Administration, Bucharest – Faculty of Psychology and Political Sciences, Teacher Training Department
Doru Ștefănescu, Social IT
Received: 16.12.2022. Accepted: 21.12.2022
© Olimpius Istrate, Simona Velea, Doru Ștefănescu. This open access article is distributed under the terms of the Creative Commons Attribution Licence CC BY, which permits unrestricted use, distribution, and reproduction in any medium, provided the original author and source are credited:
Citation:
Istrate, O., Velea, S., Ștefănescu, D. (2022). Ariadna Experiment. The Role of Artificial Intelligence in Education Sciences. Journal of Digital Pedagogy, 1(1) 15-22. Bucharest: Institute for Education. https://doi.org/10.61071/JDP.0300
____________________
Romanian version of this article:
Istrate, O., Velea, S., Ștefănescu, D. (2022). Experimentul Ariadna. Rolul inteligenței artificiale în științele educației. Revista de Pedagogie Digitală, 1(1) 15-22. București: Institutul pentru Educație. https://pedagogie-digitala.ro/experimentul-ariadna-rolul-inteligentei-artificiale-in-stiintele-educatiei/